Generative AI - The Creative Force in Artificial Intelligence
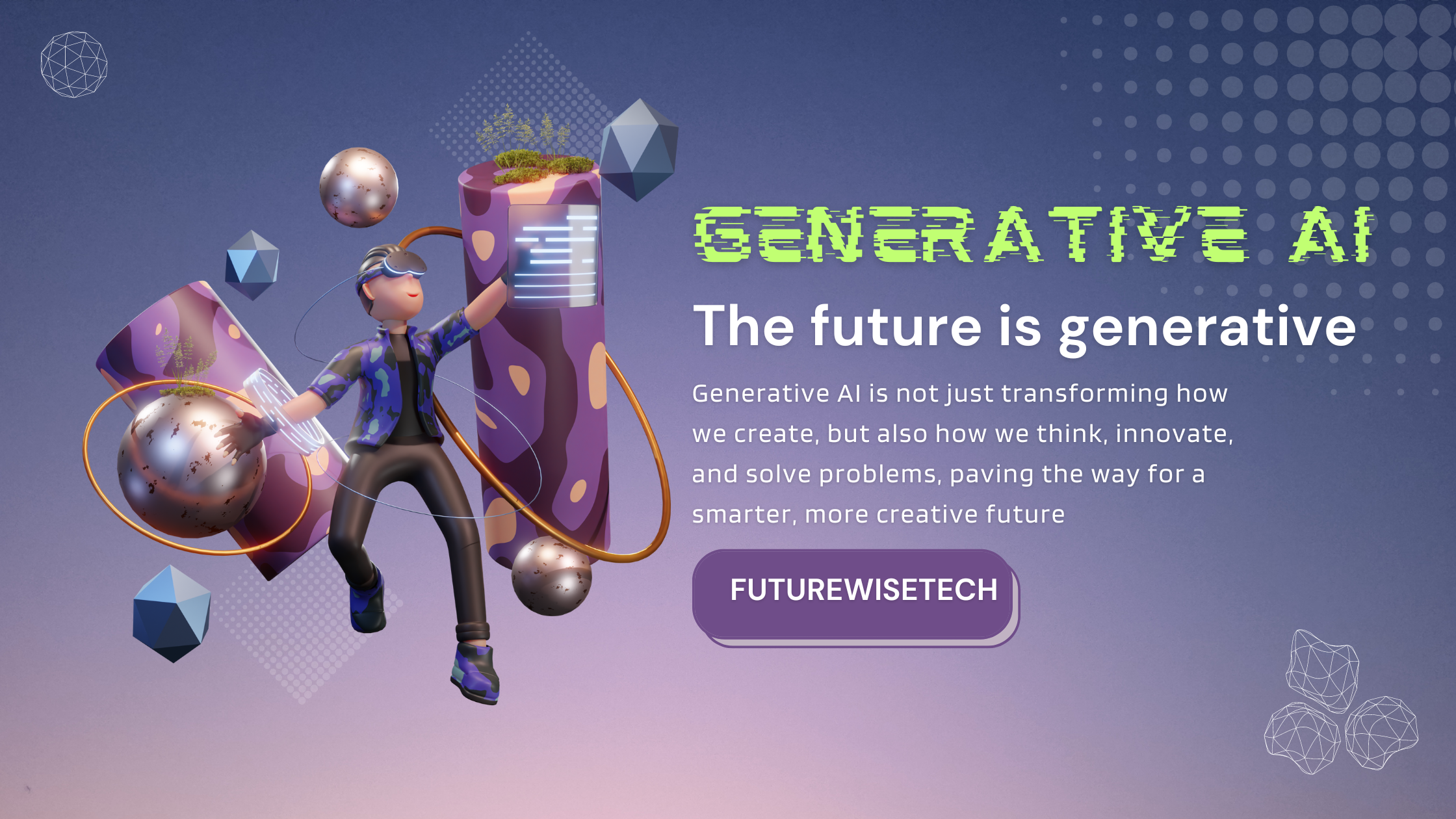
Artificial Intelligence (AI) has come a long way since its inception. Today, we're witnessing a revolution within AI, led by a fascinating subset called generative AI. But what exactly is generative AI, and how does it fit into the broader landscape of AI technologies? Let's dive in and explore this exciting field.
Understanding the AI Landscape
To grasp the concept of generative AI, it's helpful to understand its place in the AI ecosystem:
- Artificial Intelligence (AI) - The broadest category, encompassing all technologies that enable machines to mimic human intelligence.
- Machine Learning (ML) - A subset of AI that focuses on algorithms that improve through experience.
- Deep Learning (DL) - A subset of ML that uses neural networks with multiple layers.
- Generative AI - A subset of DL that creates new content based on patterns in existing data.
Generative AI sits at the cutting edge of AI technology, pushing the boundaries of what machines can create.
What is Generative AI?
Generative AI refers to AI systems that can create new content, whether it's text, images, music, or even code. These systems learn patterns from existing data and use that knowledge to generate original outputs.
Key features of generative AI include
- Creation of new, original content
- Learning from unstructured datasets
- Often using unsupervised or semi-supervised learning approaches
- Focusing on understanding the distribution of data
Types of Generative AI
There are two main categories of generative AI that have gained significant attention.
- Generative Language Models - These models, like ChatGPT, can generate human-like text. They're trained on vast amounts of text data and can produce everything from short answers to long-form content.
- Generative Image Models - These models, such as DALL-E or Midjourney, can create images from text descriptions. They've revolutionized how we think about visual creativity and design.
The Role of Large Language Models (LLMs)
Large Language Models (LLMs) play a crucial role in the world of generative AI. These are pre-trained models that have been exposed to enormous amounts of text data.

Key points about LLMs
- They're a subset of deep learning
- They overlap significantly with generative AI
- They're pre-trained on vast amounts of text data
- They can generate human-like text and perform various language tasks
Deep Learning - The Foundation of Generative AI
Deep learning, the parent category of generative AI, can be divided into two main approaches:
- Discriminative Models
- Focus on classification and prediction tasks
- Trained on labeled datasets
- Can be supervised, unsupervised, or semi-supervised
- Try to identify the relationship between input and output
- Generative Models
- Generate new data based on training data
- Often work with unstructured datasets
- Aim to understand the underlying distribution of data
Generative AI falls under the second category, focusing on creating new content rather than classifying existing data.
Applications of Generative AI
The applications of generative AI are vast and growing. Here are a few examples:
- Content Creation - Generating articles, stories, or marketing copy.
- Code Generation - Predicting code based on function names or descriptions.
- Image Creation - Generating images from text descriptions.
- Music Composition - Creating original musical pieces.
- Design - Creating new product designs or architectural layouts.
Challenges and Ethical Considerations
While generative AI offers exciting possibilities, it also presents challenges:
- Quality Control - Ensuring the generated content is accurate and high-quality.
- Originality - Balancing between inspiration and plagiarism.
- Bias - Addressing biases present in training data that may be reflected in outputs.
- Misuse - Preventing the technology from being used for harmful purposes like deepfakes or misinformation.
The Future of Generative AI
As generative AI continues to evolve, we can expect to see
- More sophisticated and nuanced outputs
- Increased integration into creative workflows
- New applications in fields like scientific research and product development
- Improved ability to understand and follow complex instructions
Conclusion
Generative AI represents a significant leap forward in artificial intelligence. By learning to create rather than just analyze, these systems are opening up new possibilities in various fields. As the technology continues to develop, it will be fascinating to see how it shapes our world and redefines the boundaries of machine creativity.
While generative AI brings exciting opportunities, it's crucial to approach its development and use thoughtfully, considering both its potential benefits and risks. As we continue to explore this technology, we must strive to harness its power responsibly, ensuring it serves to enhance human creativity and productivity rather than replace it.